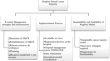
Overview
Discover Public Health, formerly Emerging Themes in Epidemiology, is an open access journal publishing research from all fields relevant to public health.
Publication costs for Discover Public Health are covered by Springer Nature until 1 January 2025. Authors whose articles are accepted for publication before that date will not pay an article-processing charge. Find out more in our submission guidelines. Current charge: £0/$0/€0 Standard charge: £1090.00/$1590.00/€1190.00
- Considers articles from a broad range of public health disciplines.
- Topics include epidemiology, occupational health, disease prevention, health policy, and more.
- A Discover journal focused on speed of submission and review, service, and integrity.
- Submission to first decision (median)
- 6 days
- Downloads
- 368,726 (2023)
Latest articles
Journal information
- Electronic ISSN
- 3005-0774
- Abstracted and indexed in
-
- BFI List
- Baidu
- CAB Abstracts
- CLOCKSS
- CNKI
- CNPIEC
- Chinese Academy of Sciences (CAS) - GoOA
- DOAJ
- Dimensions
- EBSCO
- EMBASE
- Emerging Sources Citation Index
- Gale
- Google Scholar
- Naver
- OCLC WorldCat Discovery Service
- Portico
- ProQuest
- Reaxys
- SCImago
- SCOPUS
- Semantic Scholar
- TD Net Discovery Service
- UGC-CARE List (India)
- Wanfang
- Copyright information